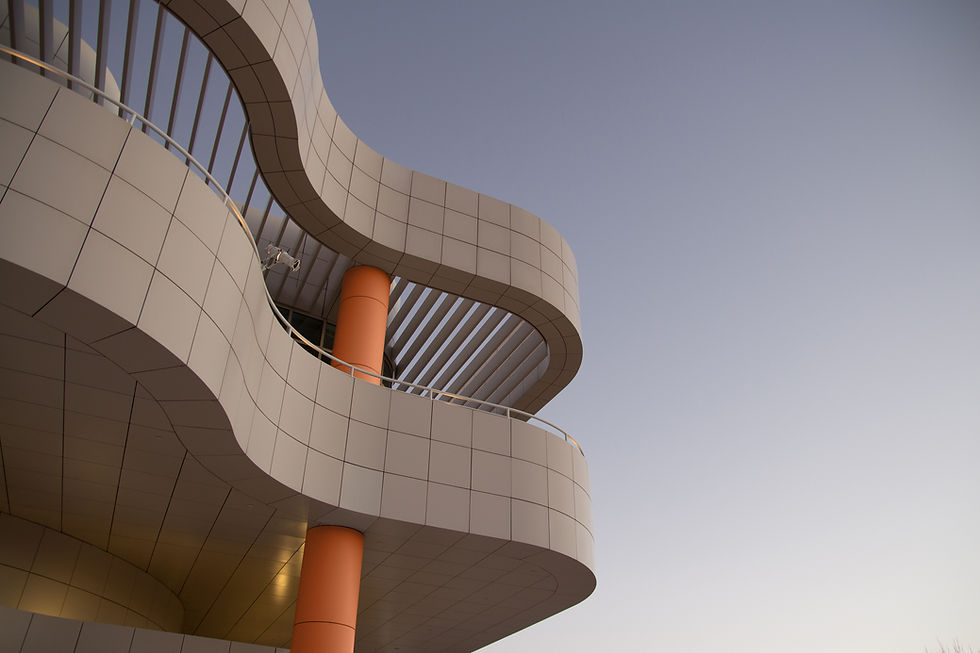
The CFO's Guide to Data Governance in the AI Era
0
6
0
Transforming Financial Leadership Through Intelligent Data Management
Executive Summary
In an era where artificial intelligence is reshaping the financial landscape, data governance has emerged as a critical success factor for modern enterprises. Recent surveys indicate that approximately 85% of Chief Financial Officers anticipate AI will fundamentally transform the finance industry within the next five years. This white paper examines the intersection of data governance and artificial intelligence in financial leadership, offering strategic insights and practical solutions for CFOs navigating this technological revolution.
Introduction
The convergence of artificial intelligence and financial management presents unprecedented opportunities for organizations to enhance decision-making, improve operational efficiency, and gain competitive advantages. However, these opportunities come with significant challenges in data management, compliance, and governance. As enterprises increasingly rely on AI-driven insights, the quality and governance of underlying data become paramount to success.
This white paper explores the current landscape of data governance in finance, examines key challenges faced by CFOs, and presents strategic solutions for implementing robust data governance frameworks in the AI era.
Section 1: The Evolution of Data Governance in Finance
1.1 The Data Revolution in Financial Management
The financial sector has witnessed a dramatic transformation in how data is collected, processed, and utilized. Traditional financial data management has evolved from simple spreadsheet-based systems to complex, interconnected ecosystems that process vast amounts of information in real-time. This evolution has created both opportunities and challenges for financial leaders.
1.2 The Role of AI in Modern Finance
Artificial intelligence has become a crucial driver of innovation in financial management, enabling:
Automated processing of financial transactions
Predictive analytics for financial forecasting
Risk assessment and management
Real-time market analysis and response
Enhanced fraud detection and prevention
Section 2: Current Challenges in Data Governance
2.1 Data Quality and Integrity
The foundation of effective financial management lies in the quality and integrity of data. Organizations face several critical challenges:
Ensuring consistency across multiple data sources
Maintaining data accuracy throughout the information lifecycle
Managing data versioning and updates
Validating data inputs and outputs
2.2 Regulatory Compliance and Security
The regulatory landscape for financial data management continues to grow more complex:
Meeting requirements of GDPR, CCPA, and other privacy regulations
Ensuring data protection across international boundaries
Managing audit trails and documentation
Implementing robust security measures
2.3 Integration and Scalability Challenges
As organizations grow, their data management needs evolve:
Integrating legacy systems with modern platforms
Scaling data infrastructure to meet growing demands
Maintaining performance while increasing data volume
Ensuring system compatibility and interoperability
2.4 Real-Time Analytics Requirements
Modern financial management demands immediate insights:
Processing high-velocity data streams
Delivering actionable insights in real-time
Maintaining accuracy under time pressure
Balancing speed with reliability
Section 3: Strategic Solutions for Modern Data Governance
3.1 Advanced Data Quality Management
Implementing robust data quality frameworks requires:
Automated data validation processes
Regular data quality assessments
Standardized data entry and processing procedures
Continuous monitoring and improvement systems
3.2 Comprehensive Compliance Solutions
Modern compliance management must include:
Automated compliance monitoring tools
Regular security audits and assessments
Documentation and reporting systems
Employee training and awareness programs
3.3 Integrated Data Management Platforms
Effective data integration requires:
Centralized data management systems
Standardized data formats and protocols
Automated data synchronization
Robust error handling and recovery procedures
3.4 Real-Time Analytics Capabilities
Implementing effective real-time analytics involves:
High-performance computing infrastructure
Advanced analytics algorithms
Automated alerting systems
Scalable processing capabilities
Section 4: Implementation Guidelines and Best Practices
4.1 Strategic Planning
Organizations should:
Assess current data governance maturity
Identify key stakeholders and requirements
Develop comprehensive implementation roadmaps
Establish clear metrics for success
4.2 Technology Selection
Consider:
Compatibility with existing systems
Scalability requirements
Total cost of ownership
Vendor support and reliability
4.3 Change Management
Focus on:
Stakeholder communication and engagement
Training and support programs
Phased implementation approaches
Regular progress assessment and adjustment
Conclusion
The transformation of finance through AI presents both opportunities and challenges for CFOs. Success in this new era requires a strategic approach to data governance that balances innovation with control, efficiency with compliance, and speed with accuracy. Organizations that implement robust data governance frameworks will be better positioned to leverage AI technologies and maintain competitive advantages in the evolving financial landscape.
About VAI Consulting
VAI Consulting specializes in helping organizations navigate the complexities of data governance and AI implementation. Our comprehensive solutions address the unique challenges faced by financial leaders in the modern business environment. For more information about how we can help your organization optimize its data governance strategy, visit www.vai-consulting.com.