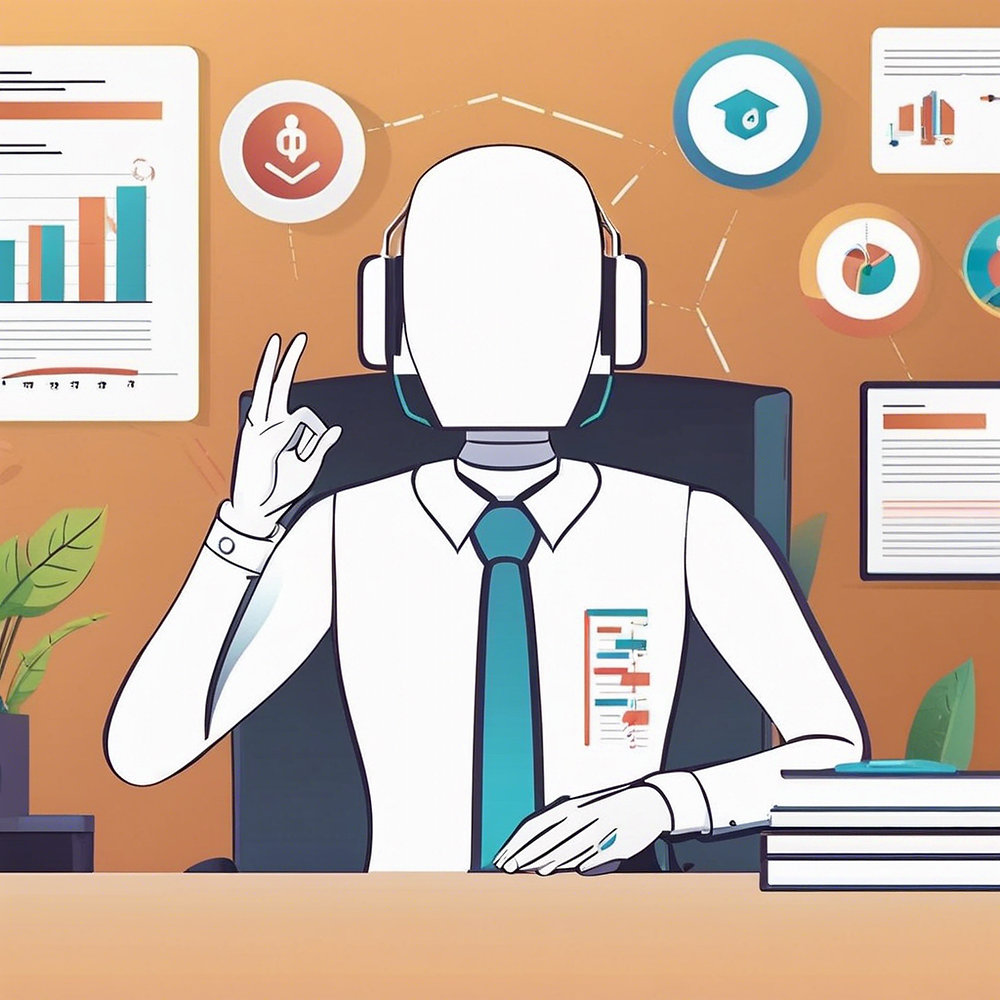
Understanding AI Agents vs. GPT-Based Automation: A Guide for Financial Executives
0
17
0
Executive Summary
Artificial intelligence is reshaping the financial sector, but executives must distinguish between true AI Agents and conventional automation workflows enhanced with large language models (LLMs) like GPT. Making this distinction is critical for informed investment decisions and avoiding costly misconceptions about AI capabilities.
Introduction
The financial technology landscape is increasingly filled with solutions marketed as "AI Agents." However, many of these solutions are traditional automation workflows enhanced with language models. This paper clarifies the differences between genuine AI agents and GPT-based automation workflows, offering financial executives a framework for evaluation and decision-making.
Defining AI Agents vs. GPT-Based Workflows
GPT-Based Workflows: The Current Reality GPT-based workflows consist of predefined operations, integration platforms, large language model APIs, and static rule-based processes. These systems excel at:
Processing structured data:Â For example, automating invoice processing by extracting data using GPT and inputting it into an ERP system.
Executing repetitive tasks:Â Such as sending templated emails or generating reports on a schedule.
Handling predictable scenarios:Â Like flagging transactions that deviate from preset thresholds in expense reports.
However, their rigidity limits adaptability. For instance, GPT workflows struggle with edge cases like interpreting nuanced policy changes or dealing with ambiguous customer queries.
True AI Agents: The Next Evolution True AI agents go beyond automation by dynamically responding to unexpected inputs, generating creative solutions, and maintaining operational continuity despite unforeseen disruptions. Key characteristics include:
Dynamic adaptability:Â AI agents can adjust to evolving business rules, such as recalibrating forecasting models when supply chain variables shift unexpectedly.
Continuous learning:Â For example, an AI Agent used for fraud detection can identify new patterns of fraudulent behavior by analyzing historical and real-time transaction data.
Autonomous decision-making:Â In portfolio management, an AI Agent can independently adjust allocations based on market conditions without waiting for human intervention.
Goal management:Â Agents can prioritize multiple objectives, such as maximizing investment returns while minimizing risk during volatile periods.
Financial Implications
Cost Considerations
Implementation Costs:
GPT-based workflows often involve minimal setup costs due to their reliance on existing platforms and APIs.
AI Agents demand higher upfront investment, including advanced infrastructure and training data preparation.
Maintenance Requirements:
GPT workflows require frequent updates to stay aligned with evolving business needs.
AI Agents are largely self-maintaining but may need occasional oversight for governance and recalibration.
ROI Analysis
Short-term Benefits:
GPT workflows offer quick wins, like automating expense reconciliations or customer service responses.
AI Agents take longer to implement but can deliver exponential returns by enabling proactive decision-making and innovation.
Long-term Potential:
GPT workflows are limited to their initial programming and require significant human intervention to scale.
AI Agents continuously improve, unlocking new efficiencies and capabilities over time.
Risk Assessment
Operational Risks:
GPT workflows are predictable but rigid, leaving organizations vulnerable to unanticipated scenarios.
AI Agents introduce complexity but offer resilience by adapting to diverse conditions.
Strategic Risks:
GPT workflows risk obsolescence as newer technologies emerge.
AI Agents require strong governance to address issues like data privacy, compliance, and ethical concerns.
Implementation Framework
Evaluation Criteria Financial executives should evaluate solutions based on:
Adaptability:Â Can the solution handle unexpected changes, such as regulatory updates?
Learning capabilities:Â Does it improve performance over time with minimal manual input?
Autonomy:Â Can it operate independently to achieve defined objectives?
Flexibility:Â Can it balance multiple priorities effectively?
Practical Examples:
A GPT workflow might automate weekly sales reporting by pulling data from a CRM and formatting it into a presentation.
An AI Agent could analyze that same sales data, identify trends, and recommend strategies to optimize pricing or inventory levels.
Red Flags Warning signs include:
Solutions that require constant manual reprogramming.
Limited handling of complex scenarios or edge cases.
Inability to adapt to new data sources or evolving requirements.
Over-reliance on static decision trees without learning capabilities.
Conclusions and Recommendations
For Immediate Implementation
Begin with defined, high-impact use cases like automating financial close processes or improving customer service efficiency.
Evaluate solutions against the criteria outlined in this paper.
Combine GPT workflows and AI Agents in hybrid models where appropriate to balance cost and capability.
For Future Planning
Monitor technological advancements in AI Agents.
Build scalable infrastructure to accommodate true AI solutions.
Develop governance frameworks that address potential risks, such as data security and compliance.
Invest in upskilling teams to effectively leverage AI tools.
Looking Forward
As technology evolves, the distinction between AI Agents and GPT-based workflows will become increasingly significant. Financial executives must stay informed, adopt flexible implementation strategies, and invest in solutions that align with long-term organizational goals. Understanding the capabilities and limitations of true AI agents is essential for making informed decisions and achieving sustained success in financial technology.